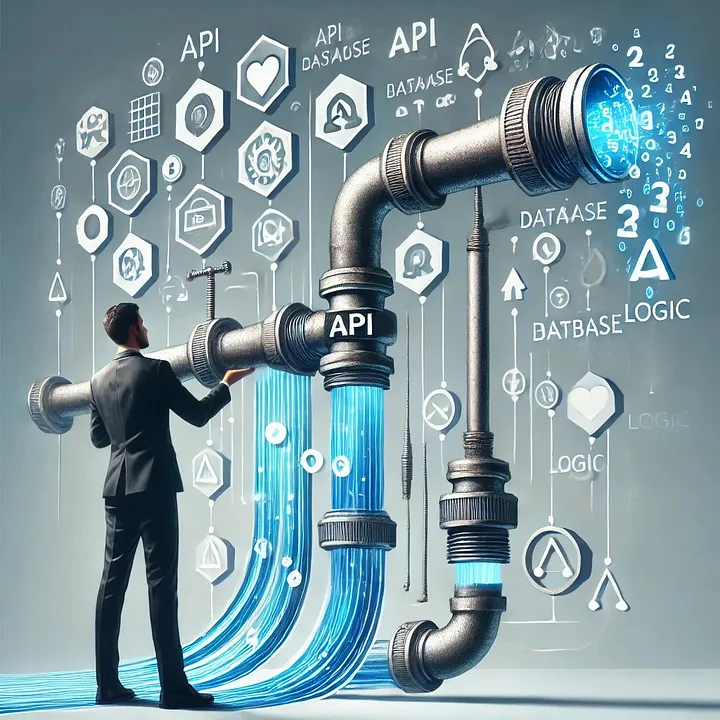
AI Product Managers Wake Up To This New Responsibility
A key difference between PM-ing traditional software products vs. AI powered offerings.
Target Audience for this article: Product Managers who wish to build AI powered offerings.
In traditional product management, data played a supportive, almost secondary role. It served two main purposes:
- Extracting Business Requirements: Data was analyzed to identify patterns or trends that would help define the product requirements. Only “valid” data points — high-quality and relevant — influenced these requirements. Any “invalid” or low-quality data was excluded or earmarked for cleanup, keeping it separate from the product’s core logic. The PM’s focus was on defining the requirements, which insulated the product from any potential data quality issues.
- Handling Data Transactions: Once the product was live, data flowed through it as user transactions, and if data quality issues arose, PMs could chalk it up to “garbage in, garbage out.” Poor data quality might impact the user’s output, but it didn’t reflect poorly on the product itself. The product’s functionality — its “plumbing” or underlying business rules — remained unaffected because these were predefined, independent of data quality.
In both cases, data quality issues were often someone else’s responsibility, whether business users, internal customers, or analytics teams. However, this paradigm shifts dramatically in AI product management.
Data as the New “Plumbing” for AI Products
In AI, the very “plumbing” — the business rules and logic of the product — is driven by data. Unlike traditional products, where a PM might define and own every business rule, AI products rely on models that learn patterns, business rules, and logic directly from data. So, rather than merely flowing through the product, data now forms the product. As a result, the quality, relevance, and structure of data directly shape how the AI model learns and behaves.
This shift places product managers at the center of data quality and sourcing, making data a primary responsibility rather than a secondary consideration. PMs are now deeply accountable for the end product’s accuracy, fairness, and performance, all of which hinge on data quality.
The Complexity of Data Sourcing
Sourcing quality data is no simple task. AI PMs must be ready to engage in detailed discussions and make strategic decisions about data quality, covering areas such as:
- Availability and Volume: Is there data available, relevant for our training? If yes, is there enough of it?
- Format and Structure: Is it in the right format, labeled correctly, and structured to fit the model’s requirements?
- Bias and Integrity: Does the data fairly represent all necessary user groups? Is it biased, or has it been compromised in any way?
- Internal vs. External Sources: Can the data be sourced internally, or does it need to be acquired? If external, what are the costs, and does it come with necessary permissions?
- Artificial Data Creation: For some scenarios of limited data, should synthetic data be created to supplement training?
These are not mere technical details; they’re strategic considerations with major implications for the product’s success and user experience. It’s why AI PMs need to develop a “muscle” for data sourcing and quality, becoming comfortable with this new, complex role. While data scientists play a significant (often primary) part in data management, the PM’s involvement is essential in defining data requirements, ensuring alignment with product goals, and making decisions that balance cost, quality, and compliance.
Quote
I once read in some article — “For AI PMs, data is no longer an input — it’s the entire blueprint.” This new responsibility requires AI product managers to think critically about data in ways traditional PMs never had to, ensuring that data truly represents the product’s purpose and user needs.
In short, if you’re managing an AI-powered product, data quality isn’t just a back-end detail; it’s the product itself. Embrace data conversations, build this new skill, and be prepared to engage deeply with data strategy.
Comments ...
No Comments Yet ...Add One