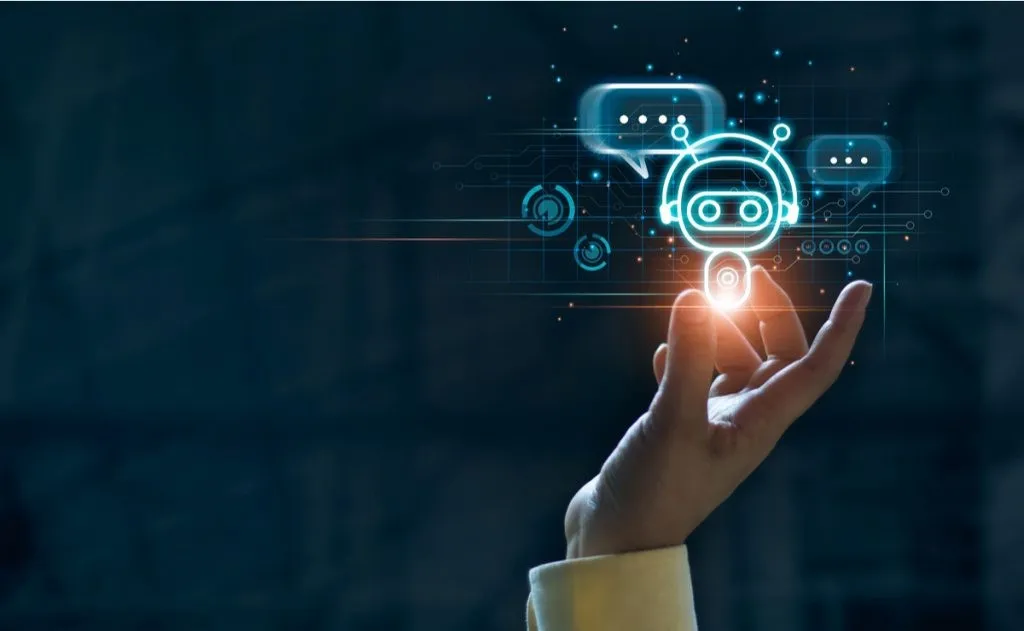
🔥A product manager’s guide to the AI Assistant (Copilot) market
What are our biggest concerns from this evolving business landscape in a Generative AI (GenAI) market worth over USD 180 billion as of 2024?
Nothing has come as close to today’s world, where we witness industries in all shapes and sizes adopt GenAI assistants — sometimes termed “Copilots” — at such a fast pace. Many professions continue to explore the current and potential impact that GenAI will have on their jobs, personal lives, and future careers. It’s both exhilarating and terrifying, and this continuous surge of adoption will only keep everyone on their toes.
One observation in this competitive landscape across both B2B and B2C software is the rise of GenAI-based assistants, otherwise branded as “Copilots” or other colloquial terms. We all know and have seen AI Assistants everywhere, whether powered by generative AI or not. We’re even talking about virtual assistants, smart home devices, and autonomous vehicles — machines that leverage predictive analytics, ML inference, and other models that don’t necessarily involve GenAI.
AI Copilots at a Glance
It’s easy to assume that the term “Copilot” refers to Microsoft’s AI product, Microsoft Copilot. However, as more organizations take an interest in leveraging AI to enhance their lines of business, the world will observe a stronger general adoption of the term for a myriad of AI-related assistants. Many examples exist, such as Planview’s Planview Copilot, Atlassian’s Rovo, Amazon Q, Cisco AI Assistant, and Notion AI.
That being said, not all AI Copilots are built the same. Many involve the use of multiple LLMs in a complete “multi-LLM” system with many AI agents at play, while others are simply based on mini models that are trained on data designed to accomplish niche use cases. If you want an idea of the typical “Copilot architecture,” it may bear some resemblance to a diagram like this:
While many AI Assistants and Copilots in today’s world leverage some variance of the above diagram, it’s important to note that this space is rapidly evolving. Tomorrow’s technologies run faster models, calibrate answers, and diversify outputs beyond just text, images, and videos.
The Market for GenAI at a Glance
The market numbers, as you could expect, are incredible. Based on Statistica, the 2024 global market for GenAI is about $184 billion, up from $135.94 billion in 2023. If we researched other sources some state that the GenAI market is projected to hit USD 1.3 trillion by 2032, such as the article by CoCreations. That’s almost as large as Mexico’s entire GDP per year.
Alongside this alarming growth, Statistica has reported that 92% of F500 companies have adopted GenAI — whether that means building their own AI assistant or adopting third-party AI tools to better their workflows.
It’s important to note — however — that the widespread organizational adoption of GenAI doesn’t necessarily mean that each entity is training its own AI models or even building its own AI Assistants; it means a company is simply leveraging some AI-powered tool (either its own or from a third-party) to complete a task at higher efficiency.
The Challenge of Discovering Use Cases
In and of itself, AI has revolutionized the tech industry simply because it’s pushed our innovative boundaries further. It’s challenged the traditional notion of unsupervised and supervised learning within the ML space and has forced many companies from the Fortune 500 into a “FOMO” state if they don’t adopt it themselves.
However, the argument is that while AI has unlocked numerous opportunities for businesses to improve their workflows:
- Those “improvements” can be hard to quantify, and
- It’s difficult to determine if a certain “use case” is the best one.
One common example could be GitHub Copilot. Many use cases are unlocked with the use of GitHub Copilot, including the automatic importing of libraries, error/bug diagnostics, general questions, writing code, and even deploying unit tests. If you ask one engineer what they use GitHub Copilot for, they might say they love it when it can autocomplete multiple lines of test methods within a code file instead of manually making the changes to each line itself. Another engineer might state that they leverage it for unit testing. Another could be using it to run diagnostics or debug code.
Determining use cases depends on several factors, including:
- The product manager's domain knowledge and/or experience to understand where AI has the highest chances of success in improving a user’s experience in their workflow.
- The ability for a product team to move and fail fast.
However, while use case discovery appears easy from a surface level, the stakes are higher when considering how expensive it is to run AI models. the 80/20 rule applies here; 80% of the value for a user derives from 20% of all use cases, but nailing down that 20% involves considerations for numerous factors, such as business viability, technical feasibility, dependencies, and of course, whether a business can differentiate itself from other competitors.
What AI Assistants must continue striving for
Given the above challenges for most product teams attempting to achieve success with AI Assistants or Copilots, I firmly believe that the best way a team can differentiate themselves in today’s market is:
- Nailing down a specific niche AI use case first. Of course, teams must double down on that said use case to become the leading competitor in the space. For example: Netlify’s AI Assistant mainly runs build error diagnostics; that’s what it does best.
- Ensure the use case doesn’t disrupt traditional user workflows. To exemplify Netlify’s AI tool again, it’s simply a button to help run diagnostics on the UI whenever a user’s build fails, without disrupting the parent UI.
- Prioritize user experience and adoption first before monetization. Netlify’s tool is free; it’s a great way to drive adoption first as the default build tool that developers can use, as users will learn of their strong AI-enhanced diagnostics to support faster error debugging.
The Future
Both the challenge of developing AI Assistants and Copilots to enhance business success, as well as predicting the future of its applications, is difficult. The market for AI use cases is always unpredictable and rapidly evolving.
But in general, I also believe that several recurring patterns will surface in the GenAI market for those consuming and producing AI products:
- Market adoption for GenAI will continue growing for the next few years, expanding opportunities for business value.
- However, eventually, the market will stabilize, resulting in a specific set of niche use cases that GenAI will replace and become the norm for.
- Content output will continue to diversify, but use cases will be niche.
- Companies should be prepared to focus more on use case adoption first before monetary gains.
It’s tough to land in that sweat spot between the three circles: landing on a “killer use case,” monetizing for business growth somehow, and ensuring the use case doesn’t disrupt traditional user workflows in your product. However, these challenges shouldn’t hinder developers from taking advantage of today’s consumer AI craze.
Given the amount of investment capital in addition to internal and external pressure, more organizations are adopting AI faster than ever before. It’s a reality that is here to stay, and companies developing AI products should stay focused on discovery and adoption.
Written by Kasey Fu
Comments ...
No Comments Yet ...Add One